The Evolving Landscape – AI’s Impact on Gap Analysis in Testing
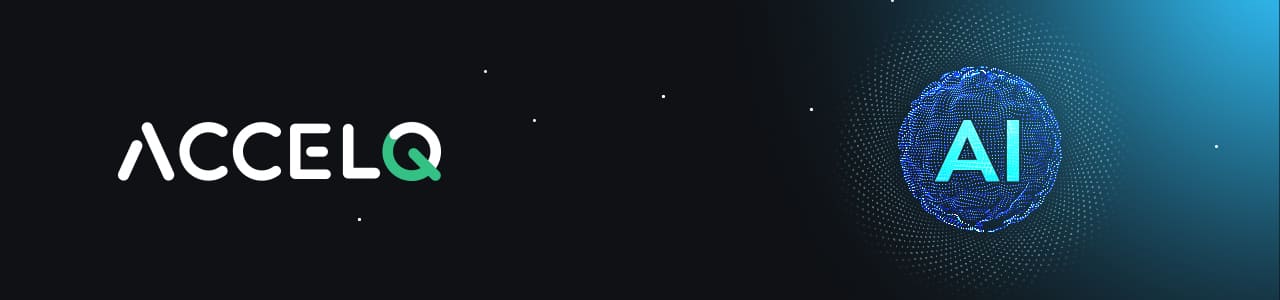
An analysis from Future Market Insights projects the AI-enabled testing tools market to reach $166.91 billion, up from $29.31 billion in 2023. The evolving AI landscape is contributing to this sharp incline. The pervasiveness of AI-powered tools in testing is, undoubtedly, a welcome sign. For this blog, we specifically focus on gap analysis in testing and how AI influences this space.
What Is Gap Analysis in Testing?
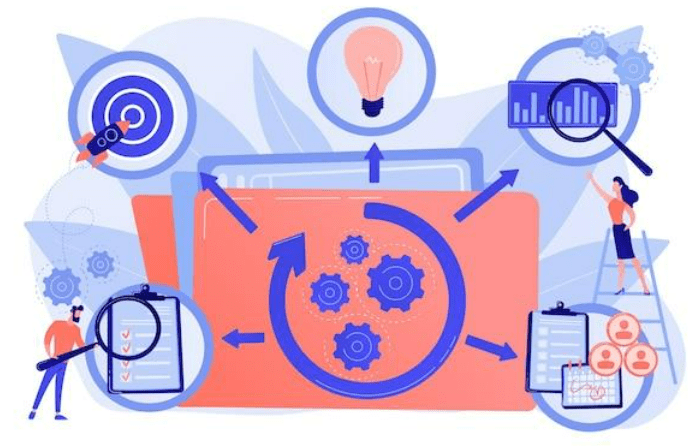
Gap analysis helps identify the difference between the current state of a software system and its desired state.
As a result, it assists in assessing performance indicators like customer acquisition, average order value, and ROI. Overall, companies adopt gap analysis to enhance the customer experience.
When talking in terms of quality assurance (QA), gap analysis is crucial in identifying discrepancies between expected and actual system performance. In essence, it ensures that software meets the desired criteria — i.e., it's high-quality and meets user needs.
How Does Gap Analysis in Testing Work?
To perform gap analysis, an analyst compares the current state of the software system to its desired state. The analyst may also generate test scenarios. After testing, the software is compared to the test scenarios to identify any gaps in coverage.
Steps Involved in Gap Analysis in Testing
Step-1: Identify System Requirements
This can be done by reviewing the system's documentation, such as the user manual, system specifications, and test plans.
Step-2: Assess the System's Current State
This involves running tests, reviewing the system's code, and talking to users.
Step-3: Identify Gaps
Upon assessing the system's requirements and current state, any gaps between the two should be identified.
Step-4: Address Gaps
The final step is to address any identified gaps, which may involve fixing defects, adding new features, or changing the system's requirements.
Do more with Test Automation
Discover more ways to add ‘low-code no-code‘ test automation in your workflows
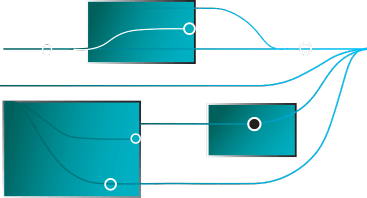
Are There Any Challenges in Performing QA Gap Analysis?
Some challenges associated with QA gap analysis include:
Defining the Scope of the Analysis
Defining the scope of a QA gap analysis can be challenging, as there are many different aspects of the QA process that could be evaluated. For that reason, it is essential to have clear goals for the analysis and focus on the areas that are most important to the business.
Gathering Data
Gathering data for a QA gap analysis can be time-consuming and challenging. Collecting data from various data sources, such as surveys, interviews, and process documentation, becomes critical to get a complete picture of the QA process.
Analyzing the Data
QA gap analysis entails analyzing the collected data, which is often complex and time-consuming. Several analytics tools might play a role in identifying the gaps.
Recommending Improvements
Once any gaps in the QA process have been identified, it is crucial to recommend specific improvements. However, these recommendations should be realistic and achievable, and they should be based on the collected data.
Implementing the Recommendations
Implementing the recommendations for improvement can be the most challenging part of the QA gap analysis process. To that end, it's critical to get a buy-in from stakeholders and train those who will implement changes.
How Can AI Influence This Process?
AI can automate the entire gap analysis process by identifying problems and recommending solutions. It's like a GPS for QA gap analysis that enables efficient and accurate testing to ensure higher-quality software. Here's how:
Analyzing Test Data and Test Cases
AI can efficiently analyze large volumes of test data and test cases and identify patterns, trends, and anomalies that may indicate gaps or areas for improvement.
Identifying Errors and Issues
AI can automatically detect errors and issues within the test data and test cases. Of course, it also eliminates the potential for human oversight or biases, ensuring more accurate and consistent results.
Finding Patterns Among Errors
AI algorithms can identify patterns among errors and determine commonalities or recurring issues. As a result, QA teams can develop a more profound understanding of the problems and pinpoint gaps in the system.
Assisting in Root Cause Analysis
Once AI has identified patterns among errors, it can help with root cause analysis. In effect, AI solutions can examine identified gaps and trace them back to their source. As a result, QA specialists can comprehend the underlying factors contributing to the issue.
Creating Comprehensive Action Plans
AI also assists in creating comprehensive action plans to address identified gaps. Through the provision of real-time, data-driven insights, QA teams can develop targeted strategies and prioritize actions that effectively mitigate gaps and improve the overall testing process.
How To Make This AI-Gap Analysis Combination Work Going Forward?
To successfully integrate AI into gap analysis for testing, it is crucial to adopt a strategic approach and consider several key factors. Here are some key considerations in that regard:
Bring the Right People Together
Organizations should focus on hiring or training professionals with expertise in AI, machine learning, data analytics, and software testing. That's because these people will be critical to creating and applying AI algorithms and models to improve the accuracy and efficiency of gap analysis.
Identify Viable Projects
Not all projects are suitable for AI-driven gap analysis. As a matter of fact, complex projects that involve large datasets or are prone to critical gaps are good candidates for AI-based testing. So, by employing AI-driven QA gap analysis in those scenarios, organizations can get the most out of this technology.
Choose the Right Tools
There are a variety of AI-powered testing tools available. When choosing a tool, it is crucial to consider the features, scalability, integration capabilities, and compatibility with existing testing frameworks. By choosing the right tool, organizations can streamline their gap analysis process and improve defect detection.
Set Time Constraints
Of course, it is important to set clear milestones and define time frames for each stage of the AI-driven gap analysis process. This will help organizations balance the need for comprehensive analysis with the need for timely delivery.
The ACCELQ Advantage for QA Gap Analysis
ACCELQ is a simple yet powerful AI-driven codeless test automation platform offering a range of testing capabilities to help businesses succeed. With it, organizations can effectively manage the QA lifecycle, accelerate the testing process, reduce maintenance efforts, lay emphasis on design and user experience, and foster profound collaboration among stakeholders.
Besides, ACCELQ's AI and ML-powered core enables significant cost savings and ushers in an innovative approach to go about QA gap analysis. Sign up for a demo here.
Geosley Andrades
Director, Product Evangelist at ACCELQ.
Geosley is a Test Automation Evangelist and Community builder at ACCELQ. Being passionate about continuous learning, Geosley helps ACCELQ with innovative solutions to transform test automation to be simpler, more reliable, and sustainable for the real world.